Algorithm & Blues: Machine-Aided Personnel Decisions Aim for Fairness, Risk Side Effects
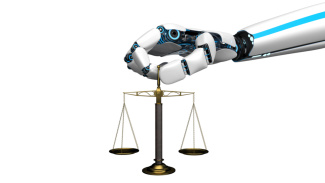
When it comes to making human resources decisions, can humans be fair? What about relying on algorithms to make decisions instead?
The answer to the first question is not always, which leads some business leaders to pursue the second. Yet, it turns out decisions made by machines are perceived as even less fair than those made by humans.
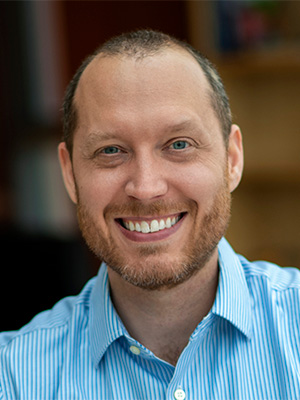
The conclusions come from a research study co-authored by Derek Harmon, assistant professor of strategy at the University of Michigan Ross School of Business. The paper was published in Organizational Behavior and Human Decision Processes, a top management and psychology journal.
Harmon, whose co-authors are David Newman and Nathanael Fast of University of California’s Marshall School of Business, discusses their research, as well as the implications as we careen ever closer toward a world where algorithms dominate our professional, personal and social spaces.
Your research finds that by taking humans out of the human resources decision-making process may reduce bias, but it’s troubling for different reasons to leave it to machines. What’s going on here?
Harmon: What’s going on is that we have a complicated set of beliefs about how machines make decisions. We believe that machines are more consistent and reliable than humans. But we also believe that machines cannot ever really “know” us, which leads us to think they cannot fairly evaluate our more intangible qualities, like character or empathy.
So what’s the solution then?
Harmon: One short-term option is to simply avoid using machines when making human-related decisions. But that’s probably imprudent. I think a longer-term alternative is that if organizations can show over time that decisions made by machines are actually fairer, it’s likely our beliefs will slowly change, too.
It seems to come down to trade-offs. I understand that’s a core theme of your work: Organizations face them and often end up creating unintended side effects in the process. What do you mean by this? And why does it happen so often?
Harmon: Organizations are made up of people, and people have lay theories about how the world works. For example, organizations are starting to use algorithms to make HR decisions because they have a lay theory about how it likely improves fairness. The problem is that even though this lay theory may sound accurate, it turns out to be wrong in many cases. This can lead to unintended outcomes.
Beyond technology, are there other examples of unintended consequences like this in your research?
Harmon: We also have lay theories about transparency. For example, we regularly see organizations making transparency pledges to signal honesty and instill confidence, but much of the time they actually create more doubt. A recent paper I published shows this in the context of Federal Reserve communications, where their efforts to be more transparent about objectives created more market volatility. It turns out when you pledge something everyone already assumes to be true, it ends up raising more questions.
That brings to mind the recent transparency pledge by COVID-19 vaccine developers “to adhere to scientific and ethical standards.”
Harmon: Exactly. This pledge might have reassured you if you thought these companies were already cutting corners. But if you assumed they were adhering to these standards all along, the pledge to abide by these standards now probably made you question if they actually weren’t before.
There’s also a growing body of research finding racial biases and other problems within facial recognition software. How does that relate to your work on human resources decision-making algorithms?
Harmon: It’s related, but I think people’s presumption about baseline fairness is different. For facial recognition software, there seems to be a prevailing belief these programs are biased, leading to a justified aversion to its use. For HR algorithms, I think it’s more complicated. At least for now, there seems to be a more widespread belief these algorithms will increase fairness by removing human bias, which makes our findings that people still dislike them all the more surprising. Of course, if we start to see consistent evidence HR algorithms are actually more biased than humans, then it’s clear this aversion to their use will be justified.
Let’s end on the broader implications for all of this. As you write in the conclusion, algorithms “gain increasing influence in human affairs.”
Harmon: I think a broader observation here is that the relationship between humans and machines is continually changing. Our paper’s findings are driven by the prevailing beliefs in today’s society. But as our beliefs about algorithms evolve alongside their use, I think we’ll see our appreciation or aversion changing, too.